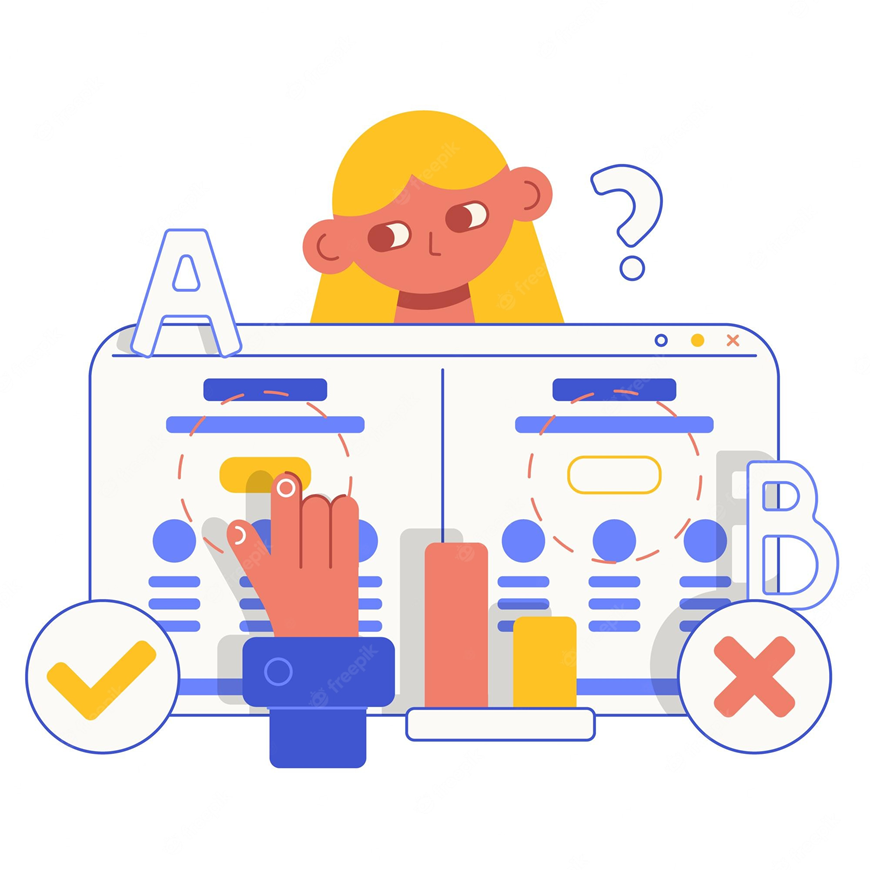
Hypothesis testing is a fundamental concept in statistics that allows us to make informed decisions and draw meaningful conclusions based on data. It serves as a powerful tool in the field of AP Statistics, enabling us to validate or reject assumptions about populations, variables, or parameters. By systematically testing hypotheses, we can gain valuable insights and make evidence-based judgments. In this comprehensive guide, we will delve into the intricacies of hypothesis testing, exploring its importance, steps, types, examples, common statistical tests, interpretation of results, and limitations.
Understanding the Concept of a Hypothesis
A hypothesis is a proposed explanation or statement about a phenomenon or relationship between variables. It serves as the foundation for hypothesis testing. In statistical terms, a hypothesis consists of two components: the null hypothesis (H0) and the alternative hypothesis (Ha). The null hypothesis represents the status quo or the absence of an effect, while the alternative hypothesis suggests a change or an effect. Hypotheses can be directional, indicating the expected direction of the effect, or non-directional, suggesting the presence of an effect without specifying its direction.
The Importance of Hypothesis Testing in Statistics
Hypothesis testing plays a crucial role in statistics as it allows us to make inferences about a population based on a sample. It helps us validate or challenge assumptions, theories, or claims, ensuring that decisions are not solely based on intuition or guesswork. Through hypothesis testing, we can determine whether observed differences or effects are statistically significant or merely due to chance. By establishing statistical significance, we can confidently draw conclusions, make predictions, or recommend actions, providing a solid foundation for decision-making in various fields, including business, medicine, social sciences, and more.
Steps Involved in Hypothesis Testing
Hypothesis testing involves a systematic process that allows us to evaluate the validity of a hypothesis. The following steps outline the general procedure for hypothesis testing:
- Formulate the Hypotheses: Clearly define the null hypothesis (H0) and the alternative hypothesis (Ha) based on the research question or problem at hand. Ensure the hypotheses are testable and mutually exclusive.
- Select the Significance Level: Choose the significance level (α) that determines the threshold for accepting or rejecting the null hypothesis. Commonly used significance levels are 0.05 (5%) and 0.01 (1%).
- Collect and Analyze Data: Gather relevant data through sampling or experimentation. Apply appropriate statistical techniques to analyze the data and calculate relevant test statistics or p-values.
- Calculate the Test Statistic: Depending on the type of hypothesis test, compute the corresponding test statistic, such as t-statistic, z-score, chi-square statistic, or F-statistic.
- Determine the Critical Region: Determine the critical region or rejection region based on the significance level and the test statistic’s distribution. This region represents extreme or unlikely results under the null hypothesis.
- Compare the Test Statistic with Critical Value: Compare the calculated test statistic with the critical value(s) to decide whether to reject or fail to reject the null hypothesis. If the test statistic falls within the critical region, reject the null hypothesis; otherwise, fail to reject it.
- Draw Conclusions and Make Inferences: Based on the decision made in the previous step, draw conclusions about the population and make inferences regarding the research question or problem. Consider the practical significance and implications of the results.
By following these steps, we can systematically and rigorously test hypotheses, ensuring a robust and reliable analysis of data.
Types of Hypotheses in Hypothesis Testing
In hypothesis testing, we encounter different types of hypotheses, depending on the research question and the nature of the problem. The two primary types of hypotheses are:
- Null Hypothesis (H0): The null hypothesis represents the absence of an effect or the status quo. It assumes that there is no significant difference or relationship between variables, and any observed differences are due to random variation or chance.
- Alternative Hypothesis (Ha): The alternative hypothesis challenges or contradicts the null hypothesis. It suggests the presence of a significant effect, difference, or relationship between variables. The alternative hypothesis can be one-sided (directional) or two-sided (non-directional), depending on whether a specific direction of the effect is expected.
Understanding the distinction between null and alternative hypotheses is vital for hypothesis testing, as it guides the decision-making process and determines the conclusions drawn from the analysis.
Hypothesis Testing Examples in Different Scenarios
To illustrate the application of hypothesis testing in various scenarios, let’s consider a few examples:
- Example 1 – Drug Efficacy: A pharmaceutical company wants to determine the efficacy of a new drug in reducing blood pressure. The null hypothesis (H0) would state that the drug has no effect, while the alternative hypothesis (Ha) would suggest that the drug significantly reduces blood pressure. By conducting a hypothesis test and analyzing the data, the pharmaceutical company can determine whether the drug has a statistically significant effect.
- Example 2 – Market Research: A marketing team wants to assess whether a new advertising campaign has a positive impact on sales. The null hypothesis (H0) would state that the advertising campaign has no effect, while the alternative hypothesis (Ha) would suggest a significant increase in sales due to the campaign. By conducting a hypothesis test and analyzing the sales data, the marketing team can evaluate the effectiveness of the advertising campaign.
- Example 3 – Educational Intervention: A school district wants to evaluate the effectiveness of a new teaching method in improving students’ math scores. The null hypothesis (H0) would state that the teaching method has no effect, while the alternative hypothesis (Ha) would suggest a significant improvement in math scores. By conducting a hypothesis test and comparing the scores, the school district can assess the impact of the teaching method.
These examples highlight how hypothesis testing allows us to make evidence-based decisions and draw meaningful conclusions in various fields.
Common Statistical Tests Used in Hypothesis Testing
In hypothesis testing, several statistical tests are commonly used, depending on the nature of the data and the research question. Some widely used statistical tests include:
- Student’s t-test: Used to compare the means of two independent groups or to assess the significance of a single sample mean.
- Chi-square test: Used to analyze the association between categorical variables or to compare observed and expected frequencies.
- Analysis of Variance (ANOVA): Used to compare the means of three or more groups to determine if there are statistically significant differences.
- Correlation analysis: Used to assess the strength and direction of the relationship between two continuous variables.
- Regression analysis: Used to model the relationship between a dependent variable and one or more independent variables.
These are just a few examples of the statistical tests available for hypothesis testing. The choice of the appropriate test depends on the research question, data type, and assumptions of the statistical test.
Interpreting the Results of Hypothesis Testing
Interpreting the results of hypothesis testing involves understanding the statistical significance, effect size, and practical importance of the findings. When the null hypothesis is rejected, it suggests that the observed results are unlikely to occur by chance alone. Conversely, failing to reject the null hypothesis implies that there is insufficient evidence to conclude a significant effect or difference.
In addition to statistical significance, the effect size measures the magnitude of the observed effect or difference. A large effect size indicates a substantial impact, while a small effect size suggests a minimal or negligible effect.
It is essential to consider the practical significance of the results, as statistical significance does not always translate into practical importance. Practical significance involves evaluating the meaningfulness or relevance of the findings in real-world contexts.
Limitations and Challenges of Hypothesis Testing
While hypothesis testing is a valuable tool in statistics, it has certain limitations and challenges that researchers need to be aware of. Some common limitations include:
- Sample Size: The size of the sample used for hypothesis testing can impact the validity and generalizability of the results. Small sample sizes may not accurately represent the population, leading to biased or unreliable conclusions.
- Assumptions: Many hypothesis tests rely on certain assumptions about the data, such as normality, independence, or equal variances. Violations of these assumptions can compromise the validity of the results.
- Type I and Type II Errors: Hypothesis testing involves the risk of making Type I errors (rejecting the null hypothesis when it is true) or Type II errors (failing to reject the null hypothesis when it is false). Researchers need to balance these errors and choose appropriate significance levels to minimize their occurrence.
- Multiple Comparisons: Conducting multiple hypothesis tests increases the likelihood of obtaining false positives. Researchers should adjust the significance level or employ correction techniques to account for multiple comparisons.
Being aware of these limitations and challenges allows researchers to make informed decisions and interpret the results accurately.
Conclusion: Harnessing the Power of Hypothesis Testing in AP Statistics
In conclusion, hypothesis testing is a powerful tool in AP Statistics that enables researchers to validate or reject assumptions, draw meaningful conclusions, and make evidence-based decisions. By understanding the concept of a hypothesis, the importance of hypothesis testing, the steps involved, types of hypotheses, examples, common statistical tests, interpretation of results, and limitations, we can harness the full potential of hypothesis testing in AP Statistics.
Whether it is evaluating the efficacy of a new drug, assessing the impact of an advertising campaign, or determining the effectiveness of an educational intervention, hypothesis testing provides a rigorous and systematic approach to data analysis. By embracing hypothesis testing, we can enhance our understanding of the world around us and make informed decisions based on evidence.
To unlock the power of hypothesis testing and enhance your statistical analysis skills, enroll in our Statistics course today! Gain a comprehensive understanding of hypothesis testing and its applications in real-world scenarios. Take your statistical analysis to new heights and make data-driven decisions with confidence.